Up today an integrated Decision Support System that combines Structural/Geotechnical and Hygro-Thermal analysis for large historic areas with climate data does not exist. These simulation tools need new and accurate sensor data to maximise the performance and the rapidity of the decision-making process; computer vision based data and artificial intelligence algorithms will be used.
In HYPERION, we will build on existing solutions and add on top further sensors, including fixed instruments within carefully selected spots in the historic areas, vehicle-based drones, forward- and side-scanning sensors, to arrive at a more comprehensive and synoptic monitoring and emergency response/damage mapping system.
The introduction of the Holistic Risk Assessment Platform as an innovative open-source planning tool that will permit quantified resilience assessment running “what-if” impact/risk/resilience assessment scenarios is also unique to the best of our knowledge. The enhanced visualisation environment, supported by wide-area surveillance and enhanced monitoring tools and existing EC services (e.g. Galileo, Copernicus) as well as the link with the existing social platform of PLUGGY to support citizens’ and stakeholders’ awareness will be one of the most innovative components of the HYPERION project.
Three pillars of innovation are included in HYPERION
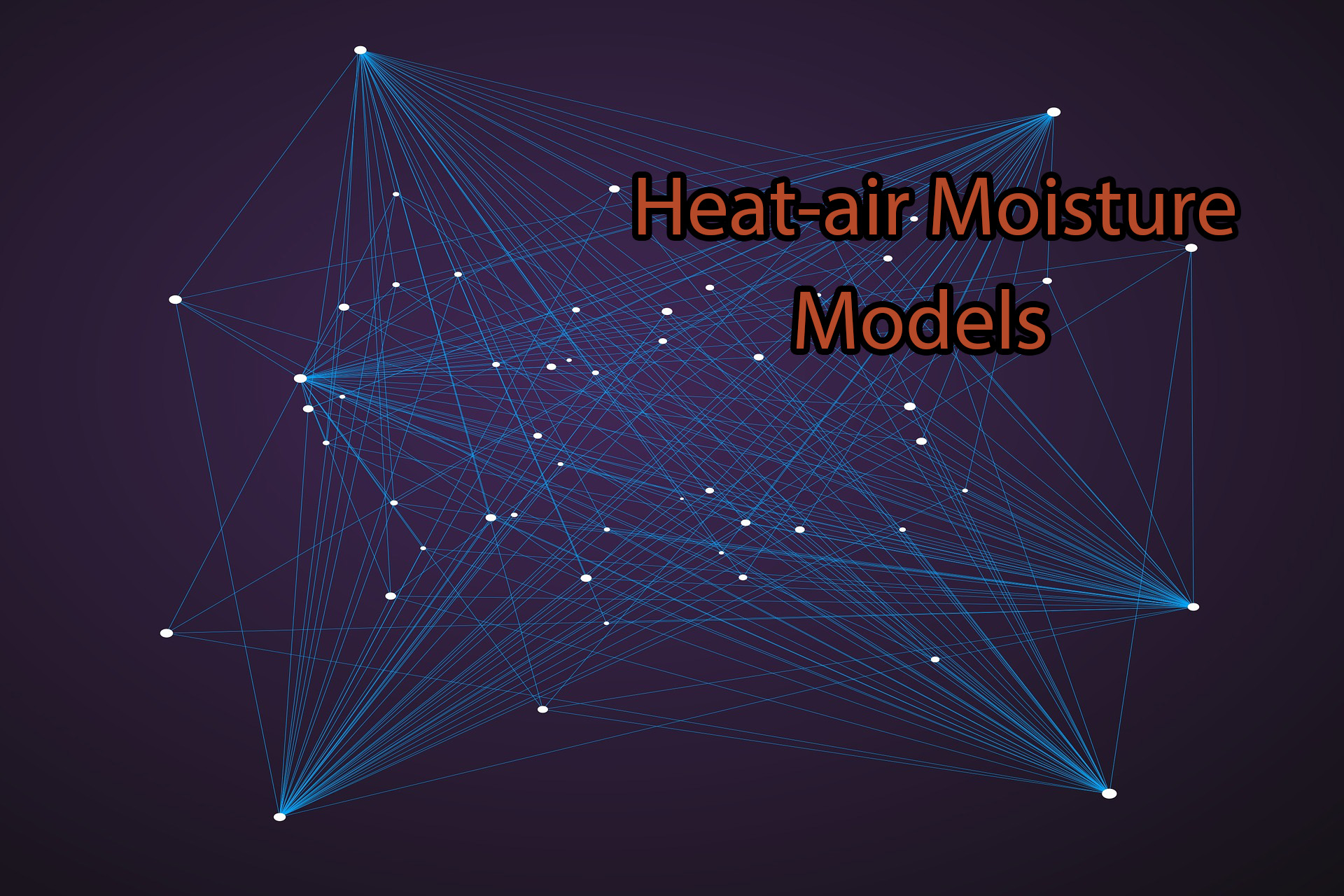
Material-specific dose-response equations for increasing dynamic Heat-Air-Moisture models accuracy
HYPERION will fill the gap of inadequacy of dose-response deterioration equations and lack in considering site-specific environmental parameters influencing future climate conditions, by improving the knowledge on measurable parameters influencing degradation rate, and refining adequate material-specific recession models to be integrated in vulnerability simulations under high resolution site-optimised climate projections.
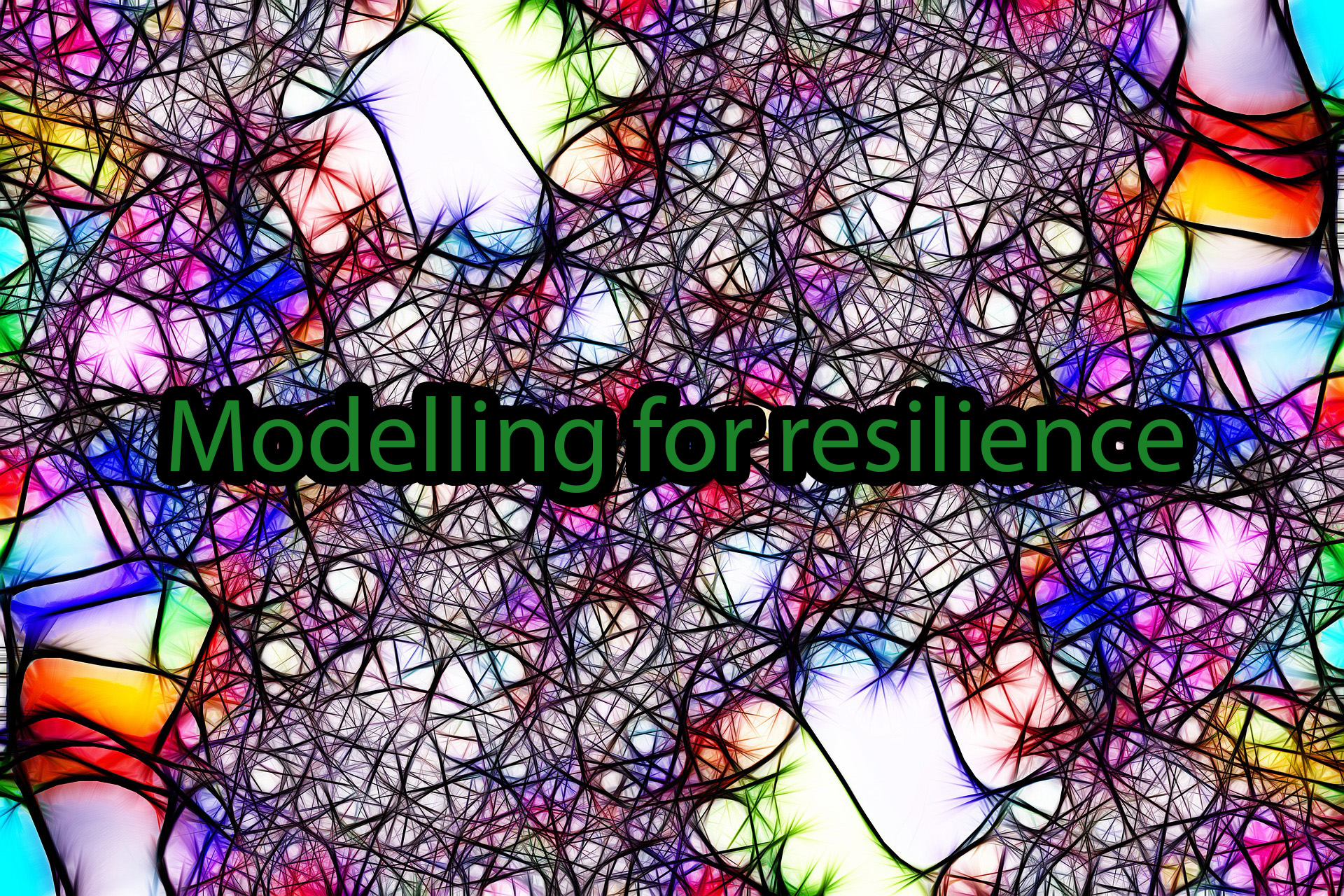
Modelling tools and simulators to be used for increasing the resilience of the historic areas
HYPERION will identify an optimal set of quantitative primary parameters and impact indicators to quantify climate change impacts on cultural heritage, encompassing climate extreme, hydrological and soil quality, and structural stress indicators. Parameter selection will consider the probability distributions of mean temperature and diurnal variation, surface hygrometry, CO2 concentrations as well as pressure inputs for wind. Uncertainties of environmental model inputs and material parameters will be quantified by uncertainty models, based on diverse sources.
New multi-scale and multi-nesting approaches for the assessment of atmospheric forcing on soil and structures will be developed to help determine the soil temperature and moisture content for different scenarios. The relevant environmental stresses yielded on the selected infrastructures under different climatic scenarios and the evaluation of the efficiency of response actions will be identified.
Downscaling of high resolution regional climate model will be performed at computational grids with horizontal resolution of a few km (0.5–3 km) in the targeted areas for specific episodes with high-impact weather and climate events. The selection of the events will be based on climate data in a way so that extreme events can be simulated and assessed.
The integration of monitoring data from advanced sensors along with the experts’ knowledge will allow for improved system identification and consequently more accurate vulnerability assessment. The availability of various scenarios stemming from high resolution regional climate model as well as multiple sources of monitoring data to be exploited by the Structural/Geotechnical simulator will allow for a more accurate prediction of the structural safety risk due to future climate change for existing structures. This will be extended to modern materials.
Modelling the function dose-response is crucial for understanding the behaviour of old materials that are not included in available databases. The range of materials and construction assemblies, as well as the variation of historical periods that the cultural heritage sites involved in HYPERION were built, will allow the creation of a materials database suitable for Heat-Air-Moisture simulations of heritage buildings and constructions. Furthermore, the HYPERION Hygro-Thermal simulation tool will include climate scenarios based on various well-documented climate change-models to predict Hygro-Thermal performance of building/ construction elements and materials degradation under these conditions.
The open-source/architecture of Holistic Risk Assessment Platform will enable an unprecedented increase in the number of available models, data, and network simulators to flow between end-users, engineers, catastrophe risk modellers, and stakeholders. Standardization and openness will allow a vibrant community to build around it, embracing existing open-source or free software and allowing inter-connectivity with proprietary simulators via standardized Application Programming Interfaces.
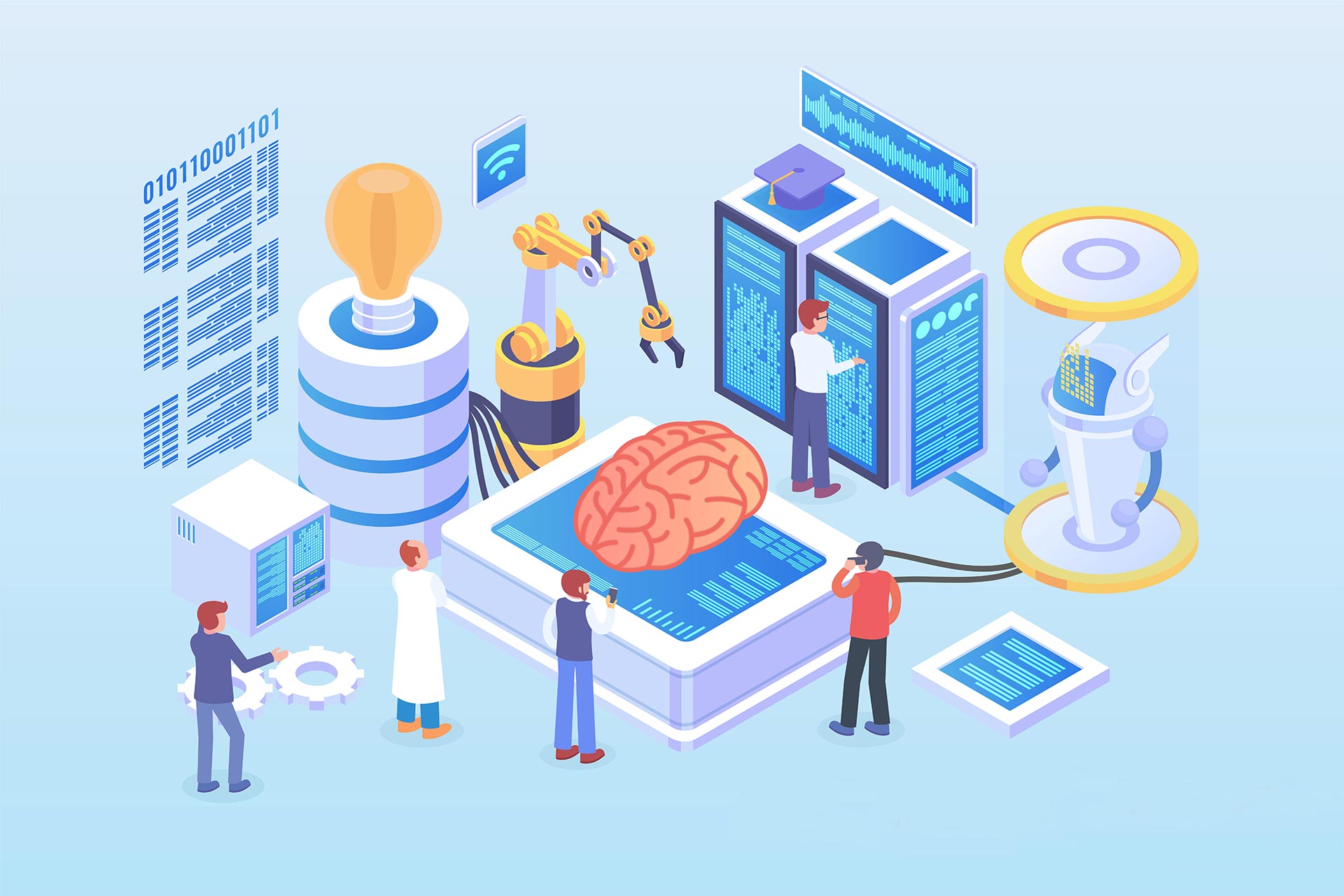
Earth Observation, Computer Vision, Machine Learning
HYPERION will integrate multi-temporal space-ground-airborne geospatial data and operational services towards monitoring hazard and exposure in near Real-Time at all geographical scales, providing evidence and supporting strategies for decision-making.
Novel technology will be developed regarding Differential Interferometric Aperture Radar methods, flood delineation and change detection and validated for analysing and delivering big earth observation data and accurate and timely geospatial information. Open data and all relevant geospatial maps and imagery will be collected offline and in near Real-Time over the pilot areas from e.g., all the Copernicus Services with both Rapid Mapping and Risk and Recovery Mapping, and the EU Flood Awareness System.
Operational Global Earth Observation System of Systems monitoring and information provision services currently developed, as well as emerging big data processing platforms will be considered.
In HYPERION, results of Robospect will be enhanced by extending the cutting-edge deep learning algorithms via an approach more targeted to cultural heritage inspection. Regarding area defects, the existing Convolutional Neural Networks -based classifiers will be initialized with Computer Vision features, as the target is mostly the precision in recognition. The flaws detection algorithms will be refined by augmenting the Convolutional Neural Networks with layers of 3D information. The processing will be on a surface development produced in the 3D information retrieval, as opposed to raw images, thus enabling 3D data extraction for structural defects.